How bad data management is hindering AI advancements.
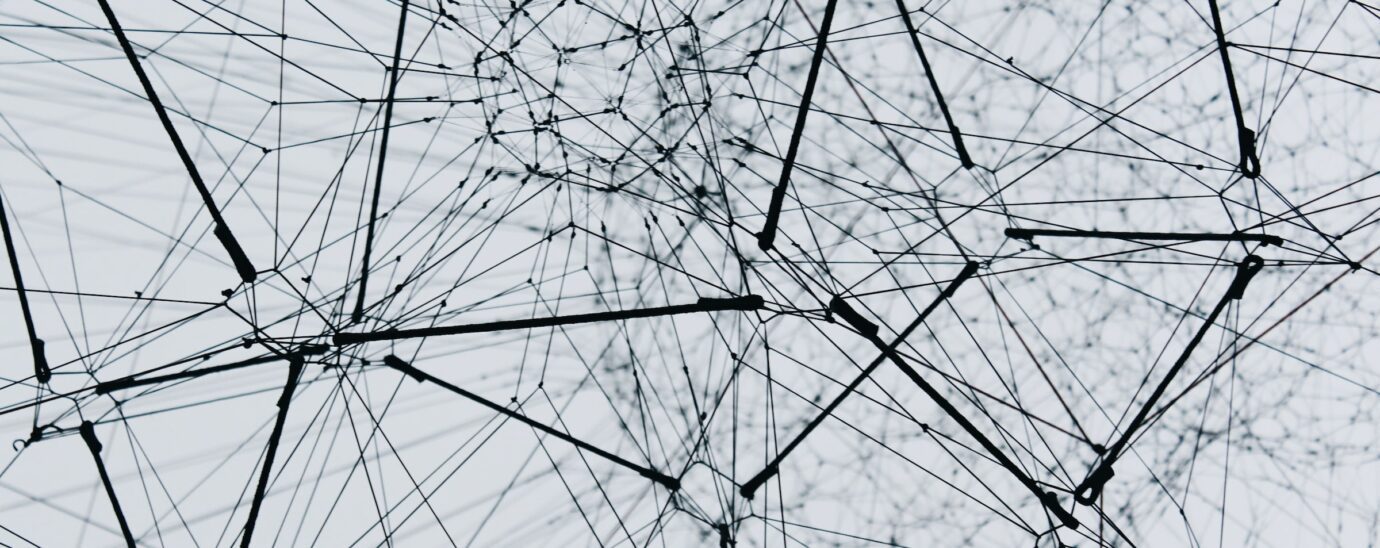
Experts conjecture that the global market for AI is set to grow 37.3 percent from 2023 to 2030. This is testament to how fundamental AI has become to steering business endeavors in the 21st century, as well as how enthusiastic those with a finger to the pulse are for increased developments. Yet many businesses are struggling to capitalize on the opportunities already offered by AI, or worse still, are unknowingly blunting their competitive edge.
The varying states of progress between AI-hungry companies is best demonstrated by the results of a recent survey of senior IT and data science professionals, conducted by Vanson Bourne. The survey revealed that 85 percent of organizations use Machine Learning (ML) or AI methodologies to build models, but only ten percent of those had been doing so for over a year. When queried on why organizations were not further ahead with AI progress, nearly half cited that data leaders within the organization were too busy with other tasks.
With 99 percent of those surveyed agreeing their data strategies had room for improvement, organizations would be wise to evaluate what is currently in place. Only then can the right steps be taken to rectify bad data and elevate insights.
AUTOMATE PIPELINES TO ELEVATE INSIGHT
To reach AI maturity, businesses need to ensure that data processes within the organization are compatible with practical applications of AI. This means, first and foremost, that data pipelines – which transport the data between applications, databases and analytics tools – must be flowing with clean, fresh and reliable data. Yet, all too often, this fundamental step is where the process breaks down, leading to bad AI insights further downstream.
When you picture the steps every business must complete to make raw data analysis-ready – including ingestion from multiple disparate sources such as business systems and applications; pre-processing; and transformation – it’s obvious that managing it completely manually, and at-scale, will create issues and waste resources. In fact, in the Vanson Bourne research, when data professionals were asked what part of the AI workflow process could be improved through automation, the two most-selected responses were data ingestion and data transformation – two steps intrinsically linked with data pipelines. A stark result when considering that 9 out of 10 businesses also claimed they still manually build and manage their data pipelines.
This approach will ultimately provide businesses with stale data that is either unusable and unreliable when fed into ML algorithms. Indeed, 71 percent of data analysts struggle to even access the data needed to run AI programs.
DEVELOPING TRUSTWORTHY DATA
This is where we find the other great barrier to successful AI adoption: distrust. When surveyed, a colossal 86 percent of respondents claimed they would struggle to trust AI to make all business decisions, because of ongoing concerns around underperforming AI models built using inaccurate or low-quality data. The process then becomes a vicious cycle, as the lack of trust complicates buy-in from stakeholders who control budgets and strategy. It’s a mistake to assume that every stakeholder will have the same practical knowledge of data processes as data teams, so communicating the negative impact of bad data is of business-critical importance. If this education is not done, the data infrastructure underpinning AI programs will continue to miss out on the attention and investment it needs to deliver material results.
Today, underperforming AI programs are costing organizations as much as five percent of their global annual revenue – in the future, this financial and opportunity cost can grow even further.
On the other hand, making simple improvements to the underlying data management processes can catalyze innovation far surpassing decision-makers’ expectations. Automating data pipeline management and centralizing data in a central location – such as a data lake – make a great place to start. By outsourcing the burden of building these systems from scratch, businesses can free up data talent to work on high value-added tasks and rest assured that data insights feeding into dashboards, reports and AI programs are clean, fresh and – crucially – reliable. Avenues for growth, that once seemed marred by hurdle after hurdle, will become obstacle free and stakeholders’ confidence in AI-led decisions will subsequently improve.
MODERNIZATION STARTS FROM WITHIN
As has always been the case, businesses cannot expect new results from old habits. With underperforming AI programs already eating into organizations’ competitive edge, now is the time to lift the veil on bad data processes and ensure all the cogs are turning smoothly.
The good news is that many businesses are already close to using their data as a springboard into AI-driven decision-making. By removing the barriers to data access and insight, they can now set their sights on the future.