Unravel Data: Watching the Big Data Throne
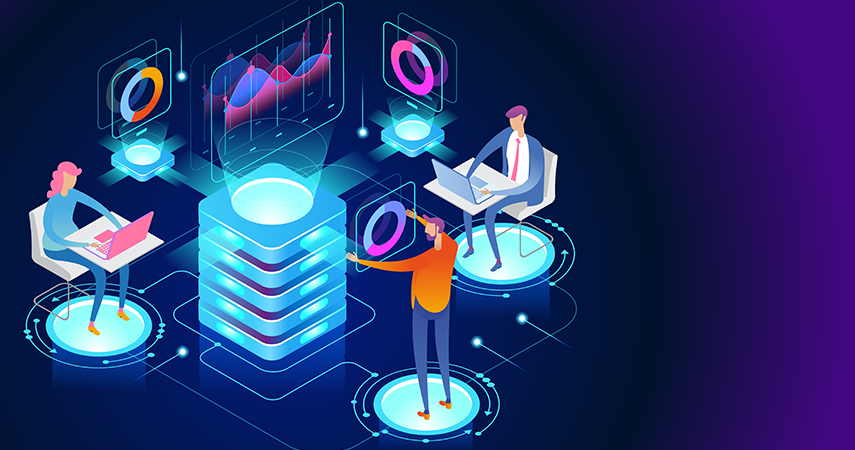
Kunal Agarwal, CEO and co-founder of Unravel Data, discusses the redefining of Hadoop’s role in the Spark and Kafka era of big data
Over the last decade, DataOps has grown exponentially more sophisticated in line with the rapid advancement of enterprise needs. With streaming data now a ubiquitous requirement within DataOps, the platform that once reigned supreme, Hadoop, has found itself increasingly marginalised by its descendants, Spark and Kafka. In the realm of big data, it is unquestionable that these newer platforms have become the de facto choice for the majority of cloud data deployments.
By providing capabilities far beyond their progenitor, Spark and Kafka are creating more value for organisations and appear to be pushing Hadoop into the fray. While the popularity of these newer platforms represents a radical shift in how enterprises deploy their DataOps, it leaves one question – what will happen to Hadoop?
The Three Great Eras of Big Data
Once the centre of countless data deployments, Hadoop is increasingly being referred to as a relic of the past or even irrelevant. However, before discussing Hadoop’s present role in the big data ecosystem, it’s necessary to see where the platform came from, how its role has changed in recent years and whether Hadoop still has any claim to the throne. To determine this, it is helpful to look at the history of big data and the three main eras that define it.
1 – Small scale big data
In its beginnings, big data was simply organisations exploring the basic functionalities of MapReduce, Pig and other native Hadoop services to see where they could create value for enterprises. Seeing as big data was still nascent, there was an extremely limited choice in technologies available to organisations. Despite this, Google, Yahoo and a small selection of other major web companies were still able to lay the groundwork for what would eventually become DataOps.
2 – Big data applications
As organisations began to recognise the possibilities of big data and the value it could generate, the technology began to see rapid development. This first manifested as the separation between storage and processing. This period is also where the cloud began to see use as an environment for data deployments – notably in Amazon EMR and Microsoft HDInsight. At the same time, Hadoop, Spark and S3 were beginning to generate value for organisations willing to invest in big data. This was primarily through basic applications like recommendation engines and fraud detection which had only recently become viable on these platforms.
3 – Advancement in adoption and sophistication of big data
The latest, and most recent, period in the big data timeline is defined by the mass-adoption of big data services. As they clearly demonstrate how they generate value for enterprises and how they can be used in increasingly specific use-cases, more enterprises are working big data into their agenda. This rapidly expanding ecosystem is being supported by newer technologies, predominantly Spark and Kafka. However, while both of these platforms are drastically reshaping the data stack, they also represent a challenge to Hadoop’s position in big data.

The Usurpers Spark and Kafka
As demand for streaming applications, data science and ML (machine learning)/ AI (artificial intelligence) continues to increase, Spark and Kafka and their roles in big data expand accordingly. Both platforms are uniquely positioned to support applications in this area and are unlikely to see competition any time soon. Spark’s unmatched speed, open-source processing and analytics engine mean that is well optimised to handle large quantities of real-time data. Likewise, Kafka offers an open-source streaming platform that is well-suited to transporting data between systems, applications, data producers and consumers. The key advantage offered by both these platforms is that they are efficient, quick, low-latency technologies geared toward leveraging streaming/real-time data.
READ MORE: Big Data – How Can Your Business Benefit?
For apps that produce or rely on a constant flow of streaming data, this is essential. Streaming data requires the rapid processing of data streams in order to extract real-time insights and encompasses common applications such as recommendation engines and IoT (Internet of Things) apps. Likewise, data science applications are increasingly using streaming data in lieu of batch data in order to provide rapid insights. Additionally, streaming data is also required for AI and ML models that aim to be constantly learning and self-training. Seeing as streaming data is integral in all these use cases, it is clear why Spark and Kafka are the de facto choice for data deployments. Until another platform can satisfy all these criteria at lower cost than Spark or Kafka, they are unlikely to see their position challenged.
That being said, Spark and Kafka both have their flaws. Primarily, debugging or tuning them can become cumbersome at scale, which is perhaps unsurprising considering that they have only recently started to offer enterprise-grade reliability at scale. Events like the ‘Spark+AI Summit’, in conjunction with efforts from the broader community, have attempted to address these issues but are yet to deliver meaningful solutions to these issues. Regardless, Spark and Kafka have rapidly come to dominate the DataOps sphere despite these drawbacks. This momentum shows no signs of stopping as more enterprises express interest in deploying their own data applications.
The Legacy of Hadoop
Seeing how prominent Spark and Kafka have become, Hadoop’s role in DataOps seems increasingly marginalised but this is not to say that it is irrelevant. Seven or eight years ago, when data deployments were as complicated as running basic BI (Business Intelligence) or database apps, Hadoop reigned supreme. While enterprise needs have changed significantly in the years since then, Hadoop still has its place.
Hadoop was more than capable when amassing data lakes was the predominant role for data deployments. However, organisations are now demanding applications that can perform far more complicated tasks than Hadoop was designed for. Platforms performing these tasks need to be able to process vast quantities of data in real-time speeds. As such, Spark was developed as a replacement for MapReduce (an older platform that wasn’t up to the task). Consequently, data teams looking to run ML, data science or streaming apps rarely consider using Hadoop when a more suitable replacement already exists.
Another consideration is that while the rise of Spark has left Hadoop out of the limelight, this is not to say that it has faded into irrelevance. Despite its limitations, there are still areas where Hadoop can outperform Spark and Kafka. For applications that need to process large quantities of data at relatively low cost, Hadoop is still one of the best choices alongside Amazon S3, Azure storage and Google Cloud storage. Likewise, Hadoop is still the obvious choice for simple data repositories.
While we tend to assume that newer technologies always eclipse their predecessors, this is not necessarily the case. Realistically, there will still be demand for the older technology as long as there still are instances where it is useful. After all, data teams won’t neglect the simpler or cheaper option simply for the sake of using the latest technologies.

The King is Dead: Long Live the King(s)
The division between Hadoop and Spark/Kafka is reminiscent of public cloud adoption. When the public cloud began trending, there was an assumption that it would make traditional data centres entirely redundant. However, the reality was that traditional data centres have specific instances where the public-cloud offers no advantage. As such, the reality of today is that the public cloud and traditional data centres enjoy a symbiotic relationship where each have their own designated and separate roles in the market. It is likely that Hadoop, Spark and Kafka will fall into a similar arrangement.
Another consideration is what the longevity of Hadoop has meant for big data teams. While Hadoop’s time in the lime-light may be coming to an end, its legacy is already emerging as the platform that originally empowered enterprises with Big Data. In this sense, Hadoop’s philosophy as an enabler of enterprise empowerment will persist, even as the platform sees less usage.
In conclusion, while Hadoop has been forced to abdicate its throne, it is likely that it will still find its own area to rule while Spark and Kafka take its former place.